
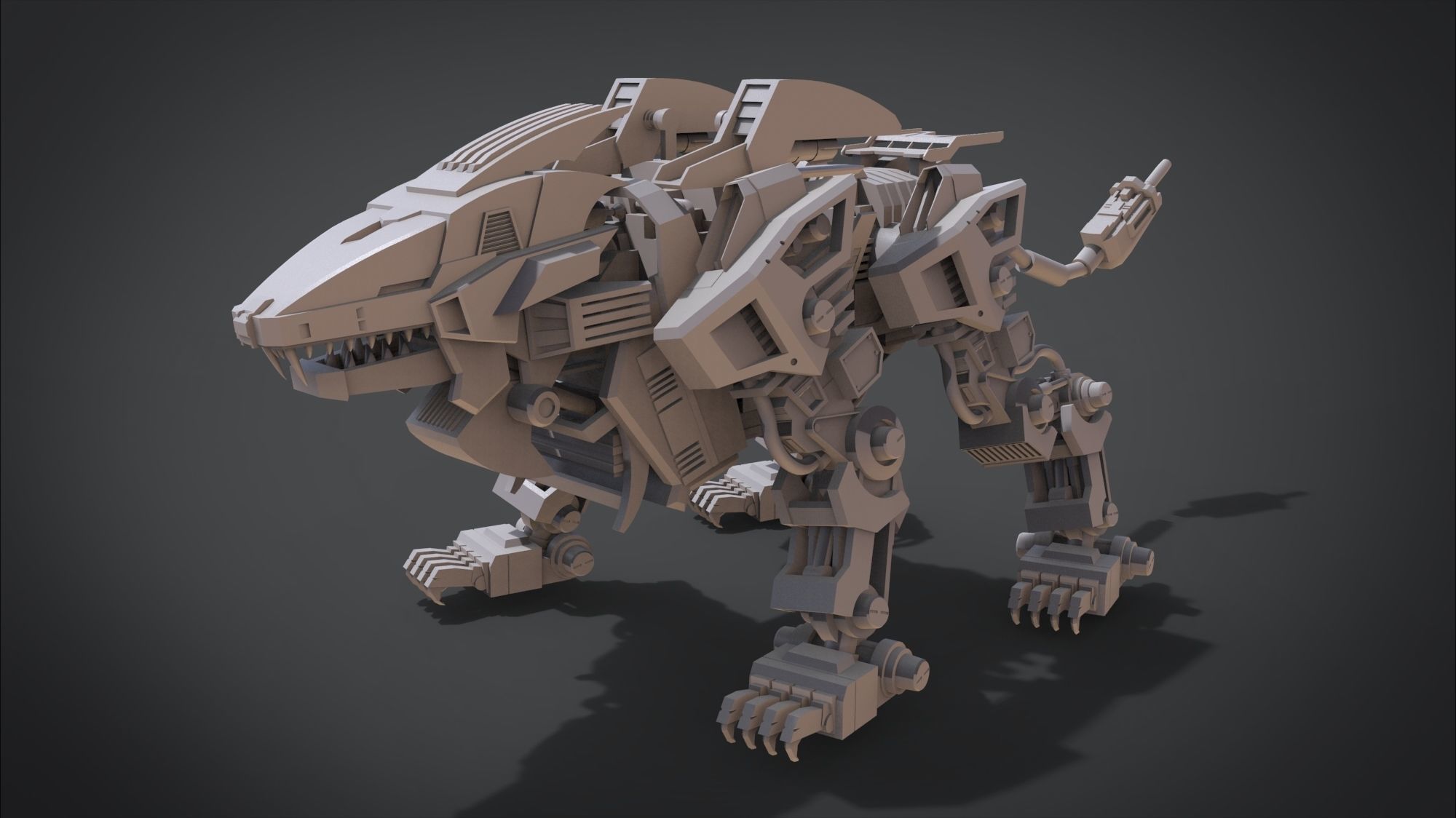
264–272 (2018)įeng, Y., Zhang, Z., Zhao, X., Ji, R., Gao, Y.: GVCNN: group-view convolutional neural networks for 3d shape recognition.

In: 2018 IEEE Conference on Computer Vision and Pattern Recognition, CVPR, pp. 2584–2591 (2013)įeng, Y., Zhang, Z., Zhao, X., Ji, R., Gao, Y.: GVCNN: group-view convolutional neural networks for 3d shape recognition. 670–676 (2018)Įlhoseiny, M., Saleh, B., Elgammal, A.M.: Write a classifier: zero-shot learning using purely textual descriptions. 30(4), 1173–1187 (2020)ĭai, G., Xie, J., Fang, Y.: Siamese cnn-bilstm architecture for 3d shape representation learning. CoRR abs/1512.03012 (2015)Ĭhi, J., Peng, Y.: Zero-shot cross-media embedding learning with dual adversarial distribution network. Extensive experiments for zero-shot 3D model classification and retrieval on two popular datasets, ModelNet40 and ShapeNetCore55, have demonstrated the effectiveness and superiority of the proposed method.Īkata, Z., Perronnin, F., Harchaoui, Z., Schmid, C.: Label-embedding for image classification. Then, we learn bidirectional projections from visual features to semantics and from semantics to visual features, which can eliminate the gap between seen and unseen domains. First, we explore the multi-view information of 3D models to construct the semantic attributes as the prior knowledge to represent 3D models. Inspired by the zero-shot learning in 2D image domain, we propose the semantically guided projection method to classify and retrieve unseen 3D models by exploring the semantic relationship between seen and unseen 3D models. How to make full use of the existing known data to represent the unknown data is a crucial topic. The most existing methods for 3D model classification and retrieval rely on the fully supervised training scheme, which are prohibitive and time-consuming to collect and label 3D models of wide different categories.
